DI-EDAI
- Contact:
- Project group:
Prof. Becker
- Funding:
BMBF
- Partner:
Karlsruher Institut für Technologie
Technische Universität München, München
Friedrich-Alexander-Universität Erlangen-Nürnberg, Erlangen
Rheinisch-Pfälzische Technische Universität Kaiserslautern-Landau, Kaiserslautern
- Startdate:
01.05.2024
- Enddate:
01.04.2027
DI-EDAI: Open source design tools for the coupled design of AI algorithms and AI chips
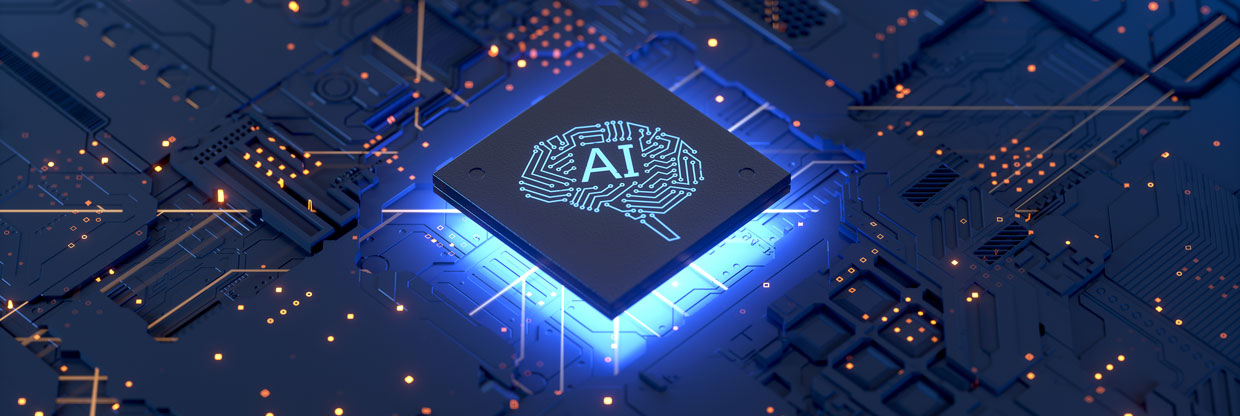
Motivation
Chip design is the essential step in designing microelectronics for specific products and applications. Competence in chip design can strengthen Germany's innovation and competitiveness and achieve a gain in technological sovereignty in Europe. In order to leverage this potential, the German and European chip design ecosystem is to be expanded. To this end, the BMBF has launched the Microelectronics Design Initiative with four key areas of focus: a strong network as a central exchange platform, training and further education for talented individuals and specialists, research projects to strengthen design capabilities and the expansion of research structures.
Project Goals
The aim of the project is to develop modern AI chips that are designed with a particular focus on security, trustworthiness and energy efficiency in various application scenarios. Another goal is to implement a seamless transition from software-based AI algorithm development to efficient hardware implementation. The focus here is on the close linking of AI and hardware in the design process as well as the development of various AI accelerators and corresponding architectures. The end result should be an automated design methodology that extends from the AI software to the AI hardware. A central aspect is the creation of an ecosystem based on open source and AI-based solutions to ensure sustainable and transparent AI system design.
ITIV Participation
ITIV is particularly concerned with securing AI hardware accelerators and architectures. This includes a fault tolerance analysis that identifies critical errors that could lead to a deviation in the AI prediction. Once the critical sources of error have been identified, targeted safety mechanisms can be implemented to increase the reliability of the system as a whole. Methods that enable extensive automation of the process will also be investigated.